Big Data Predictions: Shaping the Future of Insights
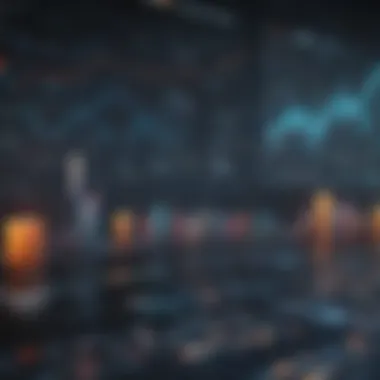
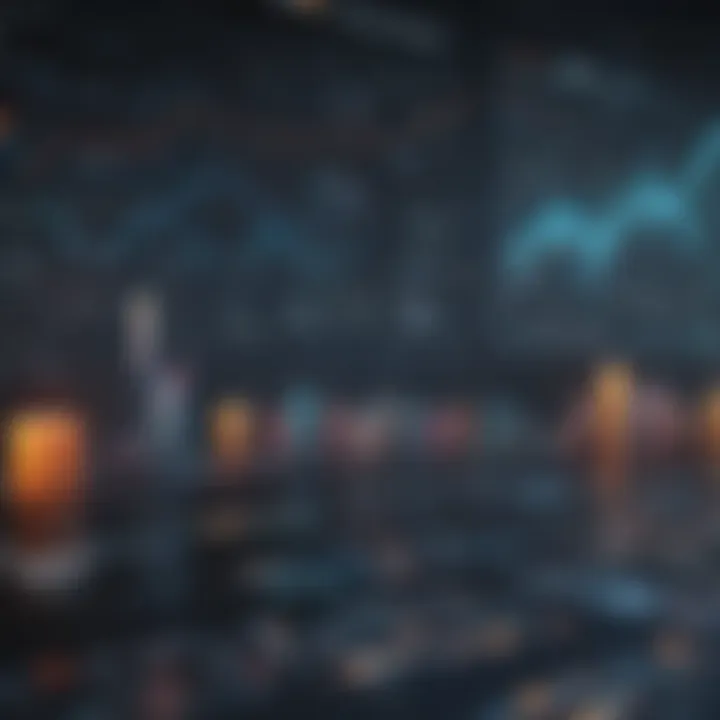
Intro
In the realm of modern businesses, data has emerged as a vital currency. The power of big data shapes decisions, strategies, and outcomes. Understanding trends and predictions tied to this evolving landscape is essential not only for large corporations but also for entrepreneurs seeking a competitive edge. Future insights will unveil hard pathways to success and prevailing challenges. As we navigate the predictive landscape of big data, entrepreneurs and business professionals alike will benefit from a detailed analysis that encompasses key developments in analytics, privacy regulations, and the influence of emerging technologies like artificial intelligence.
Entrepreneurial Insights
Entrepreneurial Success Stories
The landscape of big data is dotted with remarkable success stories. For instance, Netflix has effectively utilized big data to tailor content for individual users. This personalization stems from complex algorithms analyzing viewer habits and preferences, enabling the corporation to curate targeted suggestions. Such a data-driven approach significantly enhances viewer engagement, drastically lowering churn rates. Additionally, Amazon optimizes its product recommendations using extensive data-analysis techniques, which ultimately contributes to achieving impressive sales figures. Brands and small businesses aiming for growth would benefit from studying similar tactics employed by industry leaders.
Challenges Faced by Entrepreneurs
Despite successful narratives, obstacles exist. An overwhelming influx of data can be paralyzing. Entrepreneurs might struggle with ensuring data privacy and integration while aligning new systems with existing business structures. Implementing analytics tools may demand resources and training, leading to an uphill battle for organizations with limited budgets. An uncoordinated data strategy could ultimately backfire rather than enhance decision-making efficiency, leading to negative operational impacts.
Tips for Aspiring Entrepreneurs
- Prioritize data literacy in your team; inform your workforce about the value of data interpretation.
- Leverage open-source tools for analysis to mitigate expenses and faster implementations.
- Stay ahead of regulations to avoid potential pitfalls tied to data privacy and compliance.
- Start small by experimenting with data collection and gradually expanding your capabilities instead of overwhelming your systems.
CRM Strategies
Harnessing the power of Customer Relationship Management (CRM) systems is integral in making sense of big data. These systems support businesses in managing interactions with clients and optimizing data-driven insights for improved customer satisfaction and retention.
Importance of CRM in Business
CRM tools serve as the backbone for personalized engagement and focused customer honing. They make it easier to collect, analyze, and leverage client data. Businesses can track customer preferences, leading to tailored marketing campaigns that resonate better.
Customer insights act as the cornerstone for successful emphatic engagement strategies.
Choosing the Right CRM Software
Businesses should look for CRM software that integrates well with their existing data platforms. The B2B and B2C model require different approaches, so aiming for systems like Salesforce, HubSpot, or Zoho CRM could align more accurately with organizational needs. Features for analytics and reporting can guide decisions that will yield superior customer-based approaches deliberately.
Implementing CRM Best Practices
Successful CRM strategies must comply with fundamental procedures:
- Enhance data collection practices by identifying relevant information sources.
- Train your team around effective interface navigation and analytics extraction ways.
- Regularly assess and adjust your approaches according to outcomes transactional and customer data deliveries showcase.
Industry Trends and Analysis
Conducting thorough market analysis fosters adaptability amidst evolving consumer behavior and advancements in data technologies. Business leaders must stay sharp on trends to make informed strategic decisions.
Market Trends Impacting Entrepreneurs
Some of the buzzwords shaping upcoming trends include cloud computing, Machine Learning, and the Internet of Things (IoT). The entwining of big data with these technologies creates promising avenues for entrepreneurs truly committed to evolving past traditional business methodologies. Monitoring data trends can lead to uncovering opportunities in respective sectors, enabling competitive advantages through timely innovations.
Data-driven Insights for Successful Sales
It is essential to harness big data's predictive capabilities for better conversion rates. Utilizing analytics insights allows businesses to customize their approaches, improve efficiency in targeting leads, and refine marketing strategies tirelessly.
Case Studies and Real-life Examples
Approaching entrepreneurs with practical application provides the context necessary for understanding big data utility. When wealth management firm Wealthsimple integrated a strong CRM support system with automation, they notably increased client acquisition efficiency at minimal expense. Watching how one entity approaches big data solutions may yield inspiration, creating opportunistic pathways for other entrepreneurial aspirations.
Real-world Examples of CRM Integration
CRM integration exemplifies whether teams leverage client relationships and capitalize on relevant big data shotgun formation. Nike uses digital platforms to capture purchase behaviors, facilitating tailor-made marketing efforts tied to individual customers.
Successful Sales Campaign Case Studies
Alliance Virtual Offices has successfully adopted targeted social media strategies alongside efficient data analytics to achieve stark growth juxtaposed to less-informed campaigns across mobile platforms. This demonstrates a clear operational layout adapted through genuine customer insight based on market data.
With this foundational structure in place, we embark on a deeper exploration of the predictive elements molding the trajectory of big data as it transcends conventional frameworks, informing achievements for businesses eager for transformation.
Preface to Big Data
Big Data is changing the way organizations operate and make decisions. In this section, we focus on the fundamentals of Big Data and examine its crucial role as the backbone of modern analytics and decision-making processes. It encapsulates vast amounts of data overseen with established methodologies to turn raw numbers into valuable insights. Understanding this notion helps businesses unlock opportunities for efficiency and innovation. As the amount of available data continues to grow exponentially, there is a need for intelligent systems to process and leverage that data effectively.
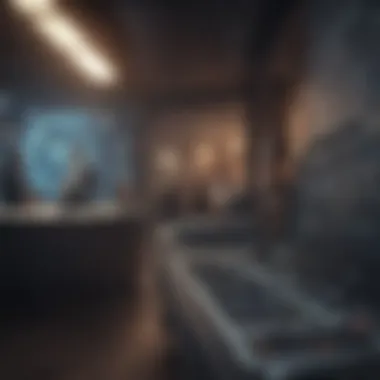
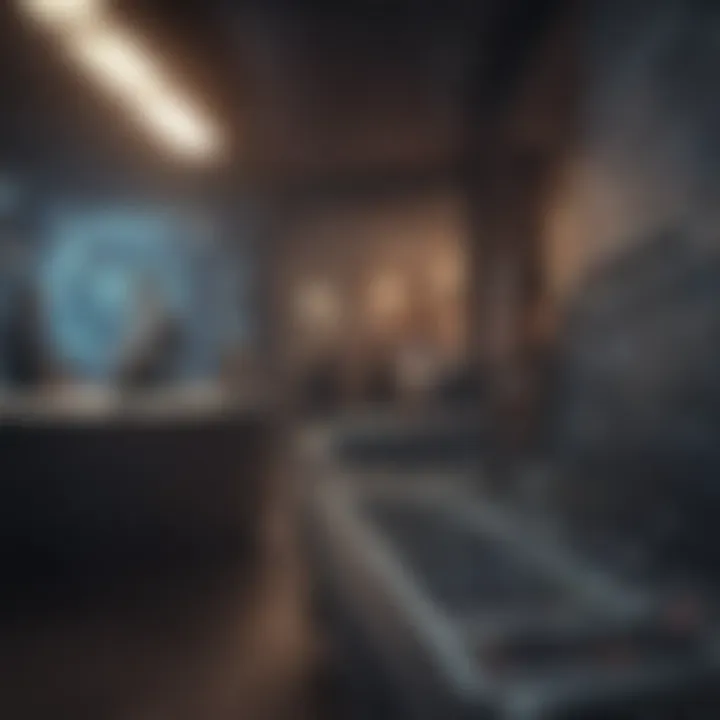
Definition and Significance
Big Data can be defined as data that is so large, fast, or complex that it is challenging to process using traditional methods. This includes structured data, such as databases, and unstructured data derived from various sources like social media, sensors, and online transactions. The significance of Big Data lies in its potential to provide actionable insights.
Practically, organizations have shifted towards data-driven strategies to enhance operations, identify trends, and improve customer interactions. Businesses can also predict future behaviors using predictive analytics powered by Big Data.
Understanding Big Data is essential
- It helps optimize processes, manage workflow,
- It ensures informed decision-making by helping build predictive models,
- Also, it provides a competitive edge in various sectors by revealing real-time trends.
Historical Context
The term
Current Trends in Big Data
Understanding current trends in Big Data is essential for businesses striving to stay competitive. The ability to manage, analyze, and utilize data effectively differentiates forward-thinking enterprises from their peers. Recognizing and adapting to these trends lays a foundation for informed decision-making and strategic growth.
Growth of Data Volume
One of the most striking trends in Big Data is the exponential growth of data volume. In a digitally-driven world, more data is generated every second than ever before. Sources like social media, IoT devices, and business transactions contribute significantly to this data deluge. For example, businesses may sit on vast customer databases cataloged by purchase history and preferences. These valuable resources can help create tailored marketing strategies that resonate directly with specific consumer segments.
However, this growth presents challenges too. Storing, processing, and analyzing such large volumes demand significant investment in infrastructure and talent. Companies need to prioritize data management strategies to transform raw information into actionable insights that can drive value.
Real-Time Data Processing
Real-time data processing has become imperative in today's fast-paced business environment. Companies are increasingly searching for instant insights that can keep them ahead of their rivals. The ability to capture and analyze data as it flows opens various possibilities. With tools such as Apache Kafka or Spark Streaming, businesses can act swiftly on trends or issues as they unfold.
This immediacy enhances operational efficiency, allowing organizations to achieve significant competitive advantages. For instance, industries like retail and finance implement real-time analytics to optimize transactions and customer experiences. E-commerce platforms are making personalized recommendations based on live consumer behavior, thus improving conversion rates. Retailers using real-time inventory tracking can streamline operations and better cater to customer demands, demonstrating the far-reaching effects of this trend.
Cloud-Based Solutions
Cloud-based solutions are revolutionizing the storage and management of Big Data. Offering scalability, flexibility, and cost-efficiency, cloud platforms are essential for many organizations handling massive data sets. Services like Amazon Web Services, Google Cloud, and Microsoft Azure provide a no-fuss, dynamic environment for data science teams.
Moreover, businesses can access powerful analytical tools and machine learning capabilities without needing extensive on-premise infrastructure. This democratizes access to advanced technologies, allowing smaller enterprises to leverage data in ways previously reserved for larger corporations. The ease of integration with other applications increases the operational benefits, as companies can now link multiple data sources efficiently.
In summary, recognizing and embracing these trends provide organizations with an opportunity to enhance their core functions while navigating the complexities associated with Big Data. This insight reflects the need for a strategic approach to data initiatives. Understanding how best to utilize vast data sets guarantees that organizations remain adaptive and responsive in an ever-evolving market landscape.
"In the realm of Big Data, insight is comparable to the guiding star for businesses."
Predicted Developments in Data Analytics
As we explore the future of big data, the topic of predicted developments in data analytics holds substantial significance. This section will delve into emerging trends and techniques set to redefine how? businesses interpret and utilize data. Enhanced analytics can enable organizations to discover deeper insights, leading to more informed, strategic decisions. Organizations that grasp these evolutions can gain a competitive edge in an era increasingly characterized by data-driven practices.
Emerging Analytical Techniques
Emerging analytical techniques represent a shift from traditional methods. Current methodologies often concentrate on descriptive analytics, but the focus is moving toward advanced techniques such as predictive and prescriptive analytics.
Some examples include:
- Predictive Analytics: This method uses historical data to forecast future events. It informs businesses about possible outcomes based on established patterns.
- Prescriptive Analytics: This approach not only predicts what is likely to happen but also recommends actions for optimal outcomes. It advises data users on what steps to take next.
- Sentiment Analysis: Businesses can benefit from opinion mining that interprets public sentiments toward their brand, helping tailor their services based on customer feedback.
Utilizing these techniques can empower organizations, allowing them to make decisions Cnformed by insights rather than intuition. This is increasingly crucial in today's data-intensive climate where timing and accuracy are paramount.
Integration with Artificial Intelligence
Artificial intelligence is becoming instrumental in advancing data analytics. The integration of AI into data practices enhances software capabilities, making analytics more agile and insightful. Key aspects of this include:
- Automated Insights: AI can swiftly proficiently process massive datasets to uncover trends and patterns. This automation allows data teams to focus on nuanced analysis rather than mundane tasks.
- Natural Language Processing: This technology promotes accessibility. Non-technical users can derive insights without needing to understand complex statistical models. Integrating NLP expands democratization of insights across an organization.
- Machine Learning Algorithms: These algorithms can learn from data. This allows analytics platforms to continually enhance performance over time, providing increasingly relevant insights.
AI's continued integration with analytics transforms Notngardian results. It can identify hidden patterns, improving existing methodologies. This integration pushes organizations toward more refined, discriminating analysis of aggregate data that drives informed decisions.
Impact of Machine Learning on Big Data
The intersection of machine learning and big data highlights a significant phase in the evolution of data analytics. With the rapid growth in data volume and complexity, machine learning presents essential tools for businesses and analysts alike. The importance of this topic lies in its potential to influence various aspects of data analysis, improving both efficiency and outcome.
Enhanced Predictive Analysis
Enhanced predictive analysis powered by machine learning enables companies to make informed decisions based on advanced pattern recognition. Traditional data analysis often relies on historical data and static models. In contrast, machine learning algorithms adapt to new data continuously, refining their accuracy. These systems condense vast amounts of data into actionable insights.
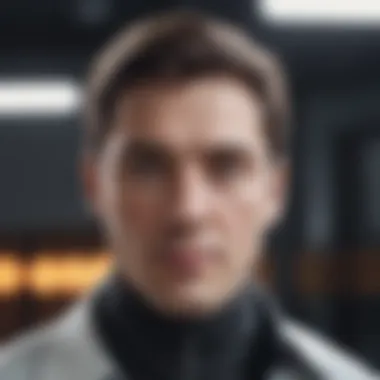
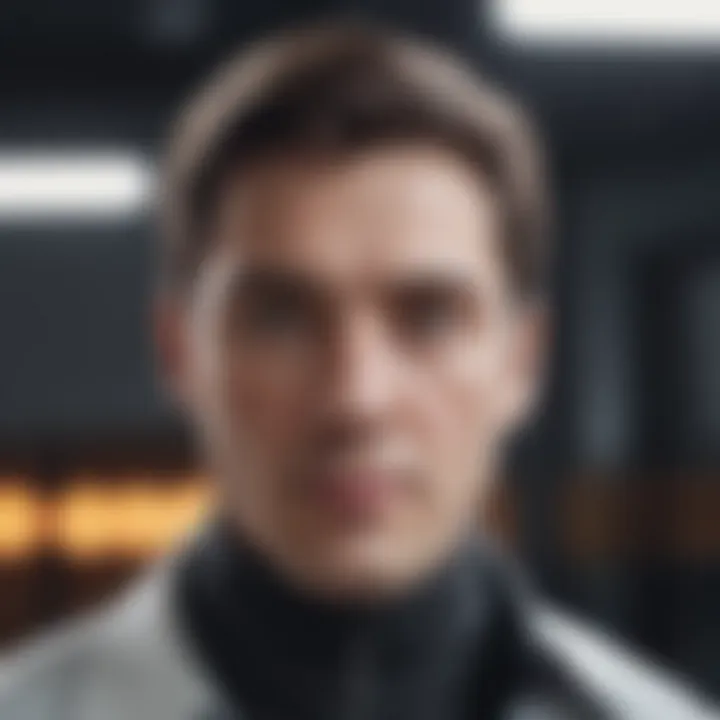
Machine learning techniques, such as regression analysis and neural networks, have opened paths to better forecasting in various industries. For example,
- Retail businesses can predict customer behavior, allowing personalized marketing strategies.
- Financial institutions can assess credit risk more accurately through algorithmic analysis.
These advancements minimize errors typically associated with human judgment in data interpretation. Anticipating future trends becomes less guesswork and more reliant on solid statistical foundation.
Moreover, organizations can tap into real-time data streams to enhance forecasting potential effectively. By integrating machine learning, businesses can shift from reactive strategies to proactive ones. Finding litmus tests, adjusting operations instantaneously, and anticipating changes in the marketplace become feasible objectives in this tech-driven methodology.
The Role of Data Privacy and Security
Data privacy and security play a crucial role in the current landscape of big data. As organizations increasingly depend on extensive datasets for informed decision-making, the safeguarding of sensitive information has transformed from a regulatory requirement to a core business objective. Effective data strategies must acknowledge the delicate balance between utilizing data insights and ensuring user trust through robust privacy measures.
Data privacy is not merely about compliance with existing laws. It is about recognizing the rights of individuals over their stored data and fostering a corporate culture that values transparency. This approach helps gain customer trust and enhances brand loyalty while mitigating the risks associated with data breaches or misuse. Security protocols must adapt to the dynamic nature of data collection practices, which continually evolve and expand.
Emerging Regulations
In recent years, several regulatory frameworks have been established worldwide. These aim to protect individuals' data while promoting accountability and transparency among organizations. The General Data Protection Regulation (GDPR) in Europe is one notable example, offering stringent guidelines about data processing and privacy rights.
Key aspects of emerging data privacy regulations include:
- Data Minimization: Organizations are encouraged to collect only the necessary data required for particular functions, reducing the risk associated with data storage.
- Consent Requirements: Clear consent processes must be established, ensuring users know how their data will be utilized.
- Right to Erasure: Individuals now often possess the right to request the deletion of their personal data, enforcing a more user-centric approach.
Many jurisdictions across the globe are expected to follow suit, as the conversation around data privacy gathers more momentum. Organizations need to stay proactive by adapting their data handling processes to comply with these evolving regulations, thereby staying ahead of potential legal challenges.
Challenges in Data Security
The scale and complexity of big data introduce inevitable challenges in ensuring data security. Despite advancements in technology, large datasets continue to be formidable targets for cyber threats.
Significant security challenges include:
- Data Breaches: The risk of unauthorized access to sensitive data can lead to significant reputational and financial repercussions.
- Insider Threats: Employees with access can inadvertently or deliberately misuse data, making robust internal policies essential.
- Evolving Threat Landscape: Cyber threats regularly adapt, making continuous monitoring and refreshment of security practices vital.
To counter these challenges, organizations must invest in advanced technologies such as encryption, behavioral analytics, and comprehensive training programs for employees. Creating a culture of security awareness within an organization also plays a critical role in establishing a secure data environment.
In today's digitized age, data privacy and security are not just organizational necessities; they are competitive differentiators.
By integrating these principles into their organizational strategies, companies can better harness the power of big data while safeguarding user trust.
Big Data in Business Intelligence
Big Data plays a pivotal role in the realm of business intelligence (BI). Understanding how to leverage large data sets can lead to informed decision-making, operational efficiencies, and enhanced competitive positioning. As organizations increasingly rely on data for strategy and outcomes, this relationship becomes ever more integral.
An important element in this domain is the way organizations utilize and analyze the vast amounts of information at their disposal. This involves understanding both the qualitative and quantitative aspects of data. Moreover, adapting to new technologies within BI can drive significant business benefits.
Utilization of Data for Decision Making
Utilizing data effectively aids in making sound decisions. Businesses have access to unprecedent amounts of data from multiple sources, including customer interactions, sales transactions, and market trends. By aggregating and analyzing these data points, organizations can identify patterns that inform their strategies.
- Real-time Insights: Immediate data analysis enables companies to respond to market demands swiftly.
- Performance Tracking: Continuous monitoring of metrics allows for timely adjustments to operations.
- Forecasting: Data analytics tools can predict future trends based on historical information, decreasing uncertainty in decision making.
Implementing strong data governance practices further supports decision-making processes such as ensuring that data is accurate, relevant, and compliant with policies.
Strategic Advantage through Analytics
Data analytics is not just a tool; it can be a strategic asset when utilized correctly. Companies that excel in data-driven cultures often outperform competitors.
- Customer Experience: Enhanced understanding of customer behavior leads to tailored marketing strategies and better customer service.
- Resource Allocation: Data helps determine where and how resources should be deployed for maximum effectiveness.
- Risk Management: Analysis aids in identifying potential risks and preparing responses proactively.
Organizations that leverage data analytics have a significant advantage over those that do not, ensuring agility and sustainability in rigid markets.
The vitality of Big Data in business intelligence cannot be contested. As technology develops, the companies that master the art of interpretation will secure a brighter future.
Industry-Specific Predictions
In the increasingly complex landscape of big data, industry-specific predictions hold immense value for maximizing insights and competitive advantages. Various sectors are leveraging the power of big data to innovate processes, improve service delivery, and drive growth. Identifying the trends that resonate uniquely in different industries should be a priority for business strategists.
Healthcare Innovations

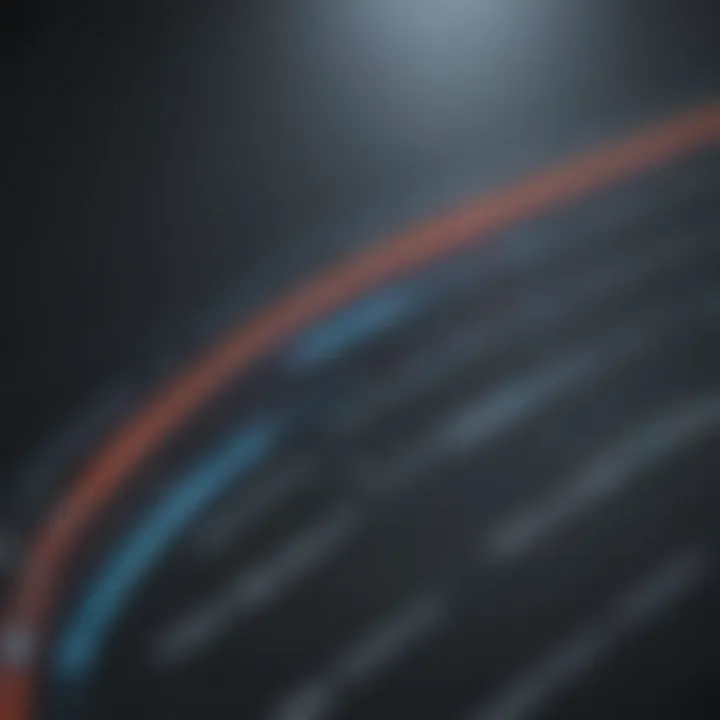
Big data in the healthcare sector is poised to revolutionize patient care. Innovative technologies collect real-time patient data from wearable devices like smartwatches or fitness trackers. This infusion of information enables healthcare professionals to improve preventative care and enhance treatment plans based on analytics. Predictive modeling is another component that actively identifies potential health risks early in a patient's life cycle.
Moreover, integrating data-driven tools into hospitals can optimize operational workflows, improving both efficiency and patient satisfaction. As the convergence of telemedicine and electronic health records enhances the speed and accuracy of diagnosis, the objective evolves further toward value-based care efforts, emphasizing outcomes over service quantity.
Retail and Consumer Insights
The retail arena is experiencing an influx of big data solutions tailored for understanding consumer behavior better than ever before. Analyzing purchasing patterns helps retailers craft personalized recommendations. Tools that harness customer sentiment analysis can adapt marketing strategies in real time, addressing any emerging issues or opportunities. The shift towards omnichannel experiences requires effective data integration from online and in-store channels to create seamless transactions for buyers.
Investments in artificial intelligence allow retailers both large and small to curate product assortments that align with customer preferences and seasonal trends. Ultimately, enhanced data utilization fosters loyalty and increases conversion rates by creating engaging experiences for both shoppers and brands.
Financial Sector Transformations
In the financial sector, big data innovations are redefining the customer journey. Banks and financial institutions adopt analytics to enhance risk management practices and streamline compliance with strict regulations. Predictive analytics can greatly aid in fraud detection by identifying irregular patterns in transactions.Tools like machine learning continuously enhance data security protocols and ensure more refined credit scoring systems which refine customer targeting outreach. The use of unique datasets, including geolocation and social media activities, allows for deeper brand interaction at pivotal moments in a consumer's lifespan.
To conclude, recognizing the industry-specific impacts of big data delves deeper into operational efficiencies and strategic decision-making. Using tailored analytics facilitates not only survival in current markets but also achieves sustainable growth. To stay ahead, it will require active adjustments toward integrating these predictive models and a continuous assessment to fully leverage opportunities pertinent to each sector.
Challenges and Limitations Ahead
The landscape of big data brings not only significant opportunities but also challenges. Recognizing these challenges is essential for businesses aiming to leverage big data effectively. As they integrate data-driven insights into their operations, the following aspects must be thoroughly evaluated:
Data Quality Concerns
Data quality is often seen as the cornerstone of meaningful insights. Poor quality data can lead to erroneous conclusions, impacting decision-making. Three aspects are prominent in discussions about data quality:
- Accuracy: Relevant data must be correct and well-refined.
- Completeness: Missing information may skew analysis.
- Timeliness: Data should be current to reflect changing conditions.
For entrepreneurs, ensuring high-quality data demands robust strategies for data collection and management. It might require specialized tools like Talend or Informatica to ensure that the data processed is both reliable and relevant.
Additionally, poor integration of data sources can lead to mismatched data points, fostering confusion further down the line. Continuous validation of data should become part of standard operations to prevent the malfunctions that stem from inadequate data quality.
Cost of Implementation
Implementing big data solutions presents considerable challenges that often center around costs. The significance of cost cannot be underestimated. Business leaders need to assess various factors when budgeting for big data initiatives:
- Infrastructure Investments: Setting up servers and database systems can be expensive.
- Software Expenses: Required analytics software adds to total costs.
- Training and Talent Acquisition: Building a skilled team or training existing employees incurs costs.
Organizations must also understand ongoing expenses such as maintenance and upgrades of their systems. These financial inputs are crucial for sustaining big data operations
Moreover, small businesses can feel particularly affected by these costs. The return on investment often takes time to materialize, leaving many decisions untapped due to fears around immediate expenditures.
In essence, identifying and comprehensively addressing these challenges lays the groundwork for successful long-term data strategies.
End: The Future of Big Data
Understanding the trajectory and future of big data is more than an academic venture; it is a vital undertaking for businesses navigating the complexities of data-driven environments. The fifth section of the article synthesizes predictions and insights on how big data will engender numerous opportunities for entrepreneurs as well as instances where caution is warranted. In grasping these developments, businesses position themselves favorably in increasingly competitive landscapes.
Synthesizing Predictions
Looking ahead, various trends in big data are anticipated to rise in prominence. Key predictions include:
- Integration of Advanced AI: The link between big data and artificial intelligence will intensify. Data analytics tools will likely become more self-sufficient because of AI algorithms, contributing to faster insights and reducing the burdens of human intervention.
- Growing Importance of Real-time Processing: Organizations will need to prioritize veracity of data inputs. Decisions made on real-time analytics may create significant competitive advantages. Data that is outdated can result in poor customer experiences.
Regular assessments of data collection methods improve30 outputs and efficiency.
Various sectors such as healthcare, finance, and retail will adapt according to these technological advancements by utilizing analytics not just for monitoring, but for predictive modeling as well. This evolution toward more nuanced interpretations of data suggests a market shift. Decisions based on predictive data guided by comprehensive analysis often exceed traditional evaluation methods.
Implications for Entrepreneurs and Businesses
The increasing reliance on big data has profound implications for the business community. Entrepreneurs crafting deals or identifying market opportunities must have a clear perspective on these crucial elements:
- Strategic Decision-Making: Data analytics will strengthen decision-making processes, not just reacting to trends but predicting movements in the marketplace. Awareness of customer preferences enabling businesses to tailor offerings can lead to better user engagement.
- Focus on Data Governance: Entrepreneurs will need to balance data collection to ensure compliance, privacy, and responsibilities. Understanding incoming regulations regarding data means adjustments might be enforced as laws evolve.
Currently, more organizations are tunning their strategies with customers at the core. Valuable insights can derive from effective usage of big data, thus they transform expressions of customer sentiments into tangible strategies for change. Outputs from big data being used in launching products or tweaking marketing strategies will compel businesses to reconsider traditional ideas about consumer engagement. Moreover, vulnerability to operational systems also arises; safeguarding data integrity must remain a constant in any transformation journey.
As big data continuously reshapes how organizations operate, this chapter on predicting its future supports the thesis that understanding these elements is crucial for achieving longevity and success in todayโs data climate.
Importance of References
- Credibility: Reliable references establish the trustworthiness of the data. Aspiring entrepreneurs and business owners can feel more confident making decisions based on well-founded information. Incorporating references from reputable sources creates a strong knowledge base.
- Diverse Perspectives: References come from various fields, providing different viewpoints on how big data affects industries and societies. This diversity allows for a holistic understanding of issues and considerations.
- Supporting Claims: Assertions about predictions and trends carry more weight when backed by substantiated sources. Whether discussing the impacts of machine learning or the regulations reshaping data privacy, utilizing relevant findings from established research strengthens arguments.
- Identifying Further Reading: For stakeholders wanting to dive deeper into specific topics, references lead to additional materials. This promotes continuous learning and informed progress in understanding the complexities of big data.
Considerations When Using References
- Reputation of Sources: Choose references from established institutions or well-known publications. Accidentally referencing unclear origins can mislead the audienceโnot something anyone wants.
- Timeliness: Given how quickly the data landscape evolves, it is important to use updated sources. Outdated data may not reflect current industrial trends, especially in technology and analytics sectors.
- Citation Formats: Follow a consistent citation style when incorporating references. This can simplify searches on specific topics for readers and enhance the readability of the document.
In sum, engaging with big data predictions without appropriate references risks misguiding the narrative, therefore diminishing its impact. For effective decision-making in today's landscape, relying on robust references enriches rather than merely supports the text. For useful resources on these topics, you might visit Wikipedia, Britannica and discussions on platforms like Reddit.